Perceptrons in artificial neural network- Artificial intelligence:
The weights in any ANN are always only real numbers and the learning problem boils down to selecting the best value for each weight in the network.
It means there are 2 essential decisions to make before we train a artificial neural network:
( i ) the whole architecture of the system ( how many hidden units/hidden layers to have, how the input nodes represent given examples and how the output information will give us an answer) and
(ii) how the units calculate their real value output from the weighted sum of real valued inputs.
The answer to (i) is typically found by experimentation with respect to the learning problem at hand: different architectures are tried and evaluated on the learning problem till the best one emerges. In perceptions, given that we have no hidden layer, the architecture problem boils down to only indicate how the input units represent the instance given to the network. The answer to (ii) is discussed in the next subsection.
Simply The input units output the value which was input to them from the instance to be propagated. Every other unit in a network usually has the similar internal calculation function that takes the weighted sum of inputs to it and calculates an output. There is different kind of possibilities for the unit function and this dictates to some extent how learning over networks of that type is performed. First, there is a simple linear unit which does no calculation; it just outputs the weighted sum which was input to it.
Second, there are other unit functions which are called threshold functions, because they are place to produce low values up until the weighted sum reaches a specific threshold, then after this threshold they produce high values. The simplest type of threshold function produces one if the weighted sum of the inputs is over a threshold value T, and produces a -1 otherwise. We call such as functions step functions, due to the reason that, when drawn as a graph, it appears like a step. Another type of threshold function is called a sigma function, which has similarity with the step function, but better over it. We will discuss at sigma functions in the next chapter.
Example
As an instance, consider a ANN which has been trained to learn the following rule categorizing the brightness of 2x2 black and white pixel images: if it contains 3 or 4 black pixels and it is dark; if it contains 2, 3 or 4 white pixels, it is bright. We may model it with perceptron by saying that there are 4 input units, one for each pixel, and they output +1 if the pixel is white and -1 if the pixel is black. The output unit also produces a 1 if the input example is to be categorized as bright and -1 if the example is dark. If we choose the weights as in the below diagram, the perceptron will perfectly categories any image of four pixels into dark or light according to our rule:
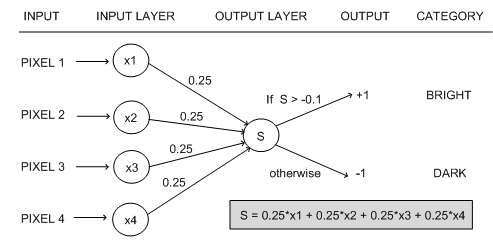
In this case we see that the output unit has a step function, with the threshold set to -0.1. In this network notice that the weights are all the similar, which is not true in the general case? It is also convenient to make the weights going in to a node add up to 1, so that it is possible to compare them simply. The reason this network absolutely captures our notion of darkness and lightness is that if 3 white pixels are input, then 3 of the input units produce +1 and one input unit produces -1. This goes into the weighted sum, giving a value of S = 0.25*1 + 0.25*1 + 0.25*1 + 0.25*(-1) = 0.5. As it is greater than the threshold of -0.1, the output node produces +1, which relates to our notion of a bright image. In the similar manner, 4 white pixels will produce a weighted sum of 1, which is bigger than the threshold, and 2 white pixels will produce a sum of 0, also bigger than the threshold. However, if there are 3 black pixels, S will be -0.5, which is below the threshold, so the output node will output -1, and the image will be categorized as dark. In the similar manner, an image with 4 black pixels will be categorized as dark. As an exercise: keeping the weights the similar, how low would the threshold have to be in order to misclassify an example with 3 or 4 black pixels?